![]() |
Testing QSUM Studies in bibliography | ![]() |
Testing QSUM
For the purposes of testing QSUM I have selected four samples of 31 sen-
tences each to determine if the samples are homogeneous or mixed utterance.
An expert QSUM practitioner has carefully processed all four samples to elimi-
nate anomalies, so I am confident that my counts of word totals are
accurate. I
have chosen to use the same test employed above, that is, two-
and three-letter
words plus words starting with a vowel. My reasons for
selecting this particu-
lar language-habit should become apparent in due
course. I identify these four
samples as W, X, Y, and Z. For each sample, I
present the relevant data in tabular
form followed by the QSUM-charts. I
adhere to Farringdon's guidelines regard-
ing scale for each of them.
As a relative newcomer to QSUM, I do not know what to make of fig-
ure 4.
Significant overlap seems to occur, but separation also occurs near the
beginning and ending of the sample. Separation in figures 5 and 6 is much
more pronounced, and I am confident that samples X and Y are mixed utter-
ances. My confidence increases on this score with sample Z. The two lines in
figure 7 criss-cross several times, a feature that Farringdon notes is
characteristic
of mixed utterance (70, 217). Also, there is only slight
overlap at the beginning
and end.
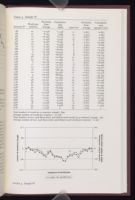
Sentence # | Words per sentence |
Deviation from average |
Cumulative sum (qsld) |
23lw+ivw | Deviation from average |
Cumulative sum (qs23lw+ivw) |
1W | 21 | −1.258 | −1.258 | 11 | −0.677 | −0.677 |
2W | 31 | 8.742 | 7.484 | 15 | 3.323 | 2.645 |
3W | 12 | −10.258 | −2.774 | 5 | −6.677 | −4.032 |
4W | 22 | −0.258 | −3.032 | 10 | −1.677 | −5.710 |
5W | 19 | −3.258 | −6.290 | 7 | −4.677 | −10.387 |
6W | 25 | 2.742 | −3.548 | 16 | 4.323 | −6.065 |
7W | 15 | −7.258 | −10.806 | 7 | −4.677 | −10.742 |
8W | 15 | −7.258 | −18.065 | 9 | −2.677 | −13.419 |
9W | 7 | −15.258 | −33.323 | 5 | −6.677 | −20.097 |
10W | 19 | −3.258 | −36.581 | 8 | −3.677 | −23.774 |
11W | 36 | 13.742 | −22.839 | 21 | 9.323 | −14.452 |
12W | 34 | 11.742 | −11.097 | 17 | 5.323 | −9.129 |
13W | 3 | −19.258 | −30.355 | 2 | −9.677 | −18.806 |
14W | 11 | −11.258 | −41.613 | 6 | −5.677 | −24.484 |
15W | 22 | −0.258 | −41.871 | 12 | 0.323 | −24.161 |
16W | 16 | −6.258 | −48.129 | 12 | 0.323 | −23.839 |
17W | 20 | −2.258 | −50.387 | 11 | −0.677 | −24.516 |
18W | 4 | −18.258 | −68.645 | 1 | −10.677 | −35.194 |
19W | 20 | −2.258 | −70.903 | 10 | −1.677 | −36.871 |
20W | 46 | 23.742 | −47.161 | 25 | 13.323 | −23.548 |
21W | 36 | 13.742 | −33.419 | 14 | 2.323 | −21.226 |
22W | 22 | −0.258 | −33.677 | 11 | −0.677 | −21.903 |
23W | 32 | 9.742 | −23.935 | 17 | 5.323 | −16.581 |
24W | 23 | 0.742 | −23.194 | 10 | −1.677 | −18.258 |
25W | 10 | −12.258 | −35.452 | 4 | −7.677 | −25.935 |
26W | 40 | 17.742 | −17.710 | 23 | 11.323 | −14.613 |
27W | 33 | 10.742 | −6.968 | 17 | 5.323 | −9.290 |
28W | 24 | 1.742 | −5.226 | 15 | 3.323 | −5.968 |
29W | 18 | −4.258 | −9.484 | 9 | −2.677 | −8.645 |
30W | 34 | 11.742 | 2.258 | 19 | 7.323 | −1.323 |
31W | 20 | −2.258 | 0 | 13 | 1.323 | 0 |
Total number of words in 31-sentence sample: 690
Average number of words per sentence: 22.258
Total number of two- and three-letter and initial-vowel words in 31-sentence sample: 362
Average number of two- and three-letter and initial vowel-words per sentence: 11.677

FIGURE 4. Sample W.
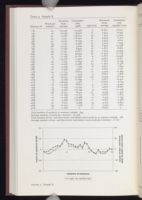
Sentence # | Words per sentence |
Deviation from average |
Cumulative sum (qsld) |
23lw+ivw | Deviation from average |
Cumulative sum (qs23lw+ivw) |
1X | 12 | −10.258 | −10.258 | 5 | −6.677 | −6.677 |
2X | 16 | −6.258 | −16.516 | 12 | 0.323 | −6.355 |
3X | 20 | −2.258 | −18.774 | 13 | 1.323 | −5.032 |
4X | 25 | 2.742 | −16.032 | 16 | 4.323 | −0.710 |
5X | 32 | 9.742 | −6.290 | 17 | 5.323 | 4.613 |
6X | 24 | 1.742 | −4.548 | 15 | 3.323 | 7.935 |
7X | 36 | 13.742 | 9.194 | 14 | 2.323 | 10.258 |
8X | 22 | −0.258 | 8.935 | 10 | −1.677 | 8.581 |
9X | 34 | 11.742 | 20.677 | 19 | 7.323 | 15.903 |
10X | 40 | 17.742 | 38.419 | 23 | 11.323 | 27.226 |
11X | 15 | −7.258 | 31.161 | 9 | −2.677 | 24.548 |
12X | 3 | −19.258 | 11.903 | 2 | −9.677 | 14.871 |
13X | 23 | 0.742 | 12.645 | 10 | −1.677 | 13.194 |
14X | 20 | −2.258 | 10.387 | 10 | −1.677 | 11.516 |
15X | 19 | −3.258 | 7.129 | 8 | −3.677 | 7.839 |
16X | 20 | −2.258 | 4.871 | 11 | −0.677 | 7.161 |
17X | 34 | 11.742 | 16.613 | 17 | 5.323 | 12.484 |
18X | 7 | −15.258 | 1.355 | 5 | −6.677 | 5.806 |
19X | 46 | 23.742 | 25 097 | 25 | 13.323 | 19.129 |
20X | 19 | −3.258 | 21.839 | 7 | −4.677 | 14.452 |
21X | 4 | −18.258 | 3.581 | 1 | −10.677 | 3.774 |
22X | 10 | −12.258 | −8.677 | 4 | 7.677 | 3.903 |
23X | 15 | −7.258 | −15.935 | 7 | −4.677 | −8.581 |
24X | 18 | −4.258 | −20.194 | 9 | −2.677 | −11.258 |
25X | 36 | 13.742 | −6.452 | 21 | 9.323 | −1.935 |
26X | 11 | −11.258 | −17.710 | 6 | −5.677 | −7.613 |
27X | 22 | −0.258 | −17.968 | 12 | 0.323 | −7.290 |
28X | 22 | −0.258 | −18.226 | 11 | −0.677 | −7.968 |
29X | 31 | 8.742 | −9.484 | 15 | 3.323 | −4.645 |
30X | 33 | 10.742 | 1.258 | 17 | 5.323 | 0.677 |
31X | 21 | −1.258 | 0 | 11 | −0.677 | 0 |
Total number of words in 31 -sentence sample: 690
Average number of words per sentence: 22.258
Total number of two- and three-letter and initial-vowel words in 31-sentence sample: 362
Average number of two- and three-letter and initial vowel-words per sentence: 11.677

FIGURE 5. Sample X.
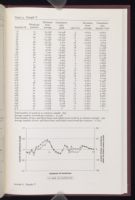
Sentence # | Words per sentence |
Deviation from average |
Cumulative sum (qsld) |
23lw+ivw | Deviation from average |
Cumulative sum (qs23lw+ivw) |
1Y | 12 | −10.258 | −10.258 | 5 | −6.677 | −6.677 |
2Y | 16 | −6.258 | −16.516 | 12 | 0.323 | −6.355 |
3Y | 34 | 11.742 | −4.774 | 17 | 5.323 | −1.032 |
4Y | 7 | −15.258 | −20.032 | 5 | −6.677 | −7.710 |
5Y | 46 | 23.742 | 3.710 | 25 | 13.323 | 5.613 |
6Y | 20 | −2.258 | 1.452 | 13 | 1.323 | 6.935 |
7Y | 25 | 2.742 | 4.194 | 16 | 4.323 | 11.258 |
8Y | 32 | 9.742 | 13.935 | 17 | 5.323 | 16.581 |
9Y | 24 | 1.742 | 15.677 | 15 | 3.323 | 19.903 |
10Y | 36 | 13.742 | 29.419 | 14 | 2.323 | 22.226 |
11Y | 19 | −3.258 | 26.161 | 7 | −4.677 | 17.548 |
12Y | 4 | −18.258 | 7.903 | 1 | −10.677 | 6.871 |
13Y | 10 | −12.258 | −4.355 | 4 | −7.677 | −0.806 |
14Y | 15 | −7.258 | −11.613 | 7 | −4.677 | −5.484 |
15Y | 18 | −4.258 | −15.871 | 9 | −2.677 | −8.161 |
16Y | 22 | −0.258 | −16.129 | 10 | −1.677 | −9839 |
17y | 34 | 11.742 | −4.387 | 19 | 7.323 | −2.516 |
18Y | 40 | 17.742 | 13.355 | 23 | 11.323 | 8.806 |
19Y | 15 | −7.258 | 6.097 | 9 | −2.677 | 6.129 |
20Y | 3 | −19.258 | −13.161 | 2 | −9.677 | −3.548 |
21Y | 36 | 13.742 | 0.581 | 21 | 9.323 | 5.774 |
22Y | 11 | −11.258 | −10.677 | 6 | −5.677 | 0.097 |
23Y | 22 | −0.258 | −10.935 | 12 | 0.323 | 0.419 |
24Y | 22 | −0.258 | −11.194 | 11 | −0.677 | −0.258 |
25Y | 31 | 8.742 | −2.452 | 15 | 3.323 | 3.065 |
26Y | 33 | 10.742 | 8.290 | 17 | 5.323 | 8.387 |
27Y | 23 | 0.742 | 9.032 | 10 | −1.677 | 6.710 |
28Y | 20 | −2.258 | 6.774 | 10 | −1.677 | 5.032 |
29Y | 19 | −3.258 | 3.516 | 8 | −3.677 | 1.355 |
30Y | 20 | −2.258 | 1.258 | 11 | −0.677 | 0.677 |
31Y | 21 | −1.258 | 0 | 11 | −0.677 | 0 |
Total number of words in 31 -sentence sample: 690
Average number of words per sentence: 22.258
Total number of two- and three-letter and initial-vowel words in 31 -sentence sample: 362
Average number of two- and three-letter and initial vowel-words per sentence: 11.677

FIGURE 6. Sample Y.
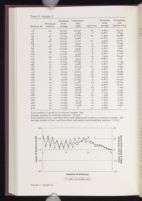
Sentence # | Words per sentence |
Deviation from average |
Cumulative sum (qsld) |
23lw+ivw | Deviation from average |
Cumulative sum (qs23lw+ivw) |
1Z | 46 | 23.742 | 23.742 | 25 | 13.323 | 13.323 |
2Z | 3 | −19.258 | 4.484 | 2 | −9.677 | 3.645 |
3Z | 40 | 17.742 | 22.226 | 23 | 11.323 | 14.968 |
4Z | 4 | −18.258 | 3.968 | 1 | −10.677 | 4.290 |
5Z | 36 | 13.742 | 17.710 | 21 | 9.323 | 13.613 |
6Z | 7 | −15.258 | 2.452 | 5 | −6.677 | 6.935 |
7Z | 36 | 13.742 | 16.194 | 14 | 2.323 | 9.258 |
8Z | 10 | −12.258 | 3.935 | 4 | −7.677 | 1.581 |
9Z | 34 | 11.742 | 15.677 | 17 | 5.323 | 6.903 |
10Z | 11 | −11.258 | 4.419 | 6 | −5.677 | 1.226 |
11Z | 34 | 11.742 | 16.161 | 19 | 7.323 | 8.548 |
12Z | 12 | −10.258 | 5.903 | 5 | −6.677 | 1.871 |
13Z | 33 | 10.742 | 16.645 | 17 | 5.323 | 7.194 |
14Z | 15 | −7.258 | 9.387 | 7 | −4.677 | 2.516 |
15Z | 32 | 9.742 | 19.129 | 17 | 5.323 | 7.839 |
16Z | 15 | −7.258 | 11.871 | 9 | −2.677 | 5.161 |
17Z | 31 | 8.742 | 20.613 | 15 | 3.323 | 8.484 |
18Z | 16 | −6.258 | 14.355 | 12 | 0.323 | 8.806 |
19Z | 25 | 2.742 | 17.097 | 16 | 4.323 | 13.129 |
20Z | 18 | −4.258 | 12.839 | 9 | −2.677 | 10.452 |
21Z | 24 | 1.742 | 14.581 | 15 | 3.323 | 13.774 |
22Z | 19 | −3.258 | 11.323 | 7 | −4.677 | 9.097 |
23Z | 23 | 0.742 | 12.065 | 10 | −1.677 | 7.419 |
24Z | 19 | −3.258 | 8.806 | 8 | −3.677 | 3.742 |
25Z | 22 | −0.258 | 8.548 | 12 | 0.323 | 4.065 |
26Z | 20 | −2.258 | 6.290 | 13 | 1.323 | 5.387 |
27Z | 22 | −0.258 | 6.032 | 11 | −0.677 | 4.710 |
28Z | 20 | −2.258 | 3.774 | 10 | −1.677 | 3.032 |
29Z | 22 | −0.258 | 3.516 | 10 | −1.677 | 1.355 |
30Z | 20 | −2.258 | 1.258 | 11 | −0.677 | 0.677 |
31Z | 21 | −1.258 | 0 | 11 | −0.677 | 0 |
Total number of words in 31-sentence sample: 690
Average number of words per sentence: 22.258
Total number of two- and three-letter and initial-vowel words in 31-sentence sample: 362
Average number of two- and three-letter and initial vowel-words per sentence: II.677

FIGURE 7. Sample Z.
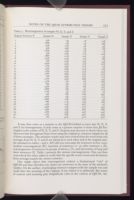
Original Sentence # | Sample W | Sample X | Sample Y | Sample Z |
1 | 3W | 1X | 1Y | 12Z |
2 | 16W | 2X | 2Y | 18Z |
3 | 12W | 17X | 3Y | 9Z |
4 | 9W | 18X | 4Y | 6Z |
5 | 20W | 19X | 5Y | 1Z |
6 | 5W | 20X | 11Y | 22Z |
7 | 18W | 21X | 12Y | 4Z |
8 | 25W | 22X | 13Y | 8Z |
9 | 7W | 23X | 14Y | 14Z |
10 | 29W | 24X | 15Y | 20Z |
11 | 11W | 25X | 21Y | 5Z |
12 | 14W | 26X | 22Y | 10Z |
13 | 15W | 27X | 23Y | 25Z |
14 | 22W | 28X | 24Y | 27Z |
15 | 2W | 29X | 25Y | 17Z |
16 | 27W | 30X | 26Y | 13Z |
17 | 31W | 3X | 6Y | 26Z |
18 | 6W | 4X | 7Y | 19Z |
19 | 23W | 5X | 8Y | 15Z |
20 | 28W | 6X | 9Y | 21Z |
21 | 21W | 7X | 10Y | 7Z |
22 | 4W | 8X | 16Y | 29Z |
23 | 30W | 9X | 17Y | 11Z |
24 | 26W | 10X | 18Y | 3Z |
25 | 8W | 11X | 19Y | 16Z |
26 | 13W | 12X | 20Y | 2Z |
27 | 24W | 13X | 27Y | 23Z |
28 | 19W | 14X | 28Y | 28Z |
29 | 10W | 15X | 29Y | 24Z |
30 | 17W | 16X | 30Y | 30Z |
31 | 1W | 31X | 31Y | 31Z |
It may thus come as a surprise to the QSUM faithful to learn that W, X, Y,
and Z are homogeneous. It may come as a greater surprise to learn that Jill Far-
ringdon is the author of W, X, Y, and Z. Surprise may increase to shock when
one
discovers that throughout I have been using the original 31-sentence
sample for all
of these examples. The attentive reader may have noticed that
the word totals and
averages from W, X, Y, and Z are identical to each other
and to the original sam-
ple tabulated in tables 1 and 2. All I did was
rearrange the sentences in four ways:
random rearrangement (W), insertion of
sentences 17–30 after sentence 2 (X),
rearrangement of sentences in
groups of about 5 (Y), and alternation of long and
short sentences (Z).
Table 7 presents the various rearrangements. One can then
cross-check the
other tables to verify that the specific word counts and deviations
from
averages match the correct sentence.
One might object that rearrangement violates a fundamental "rule" of
QSUM
and that therefore my charts are irrelevant to the issue of the method's
validity. On the surface, rearranging sentences tampers with the sample text and
could alter the meaning of the original, if not reduce it to gibberish. But
issues
of content and meaning play insignificant roles in the context of
QSUM. Ad-
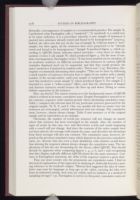
I performed what Farringdon calls a "sandwich": "A 'sandwich' is a useful test:
as its name indicates, it is a procedure whereby a new sample of sentences is
inserted into utterance already tested and found to be homogeneous" (305n14).
Indeed, she often uses this test. In the case of sample X, I did not insert a "new"
sample, but then again, all the sentences here were purported to be "already
tested and found to be homogeneous." Sample X produced figure 5, which ac-
cording to QSUM clearly indicates mixed utterance—even more so than the
random version I call sample W, which produced figure 4. On the issue of ran-
dom rearrangement, Farringdon writes: "It has been pointed out by members of
an academic audience on different occasions that sentences in various QSUM
examples displayed need not be sequential, and that they would produce the
same consistency if analysed in random order. This is true" (114). Elsewhere, Far-
ringdon recommends alternating short samples: "This can be done by following
a small number of sentences (between four to eight) of one author with a similar
number of the second author, until your sample is completely used up" (120). I
used this method to create sample Y, which produced figure 6. For sample Z, I
intended to create a "roller-coaster" effect, such that the alternation of longest
and shortest sentences would bounce the lines up and down. Doing so causes
definite separation in this instance.
How can this be? The answer returns us to the fundamental nature of QSUM
which is evident in its name: cumulative sums. Despite Farringdon's assurances to
the contrary, sequence order matters greatly when calculating cumulative
sums.
Table 7 compares the relevant data for any particular sentence
generated for the
original sample, W, X, Y, and Z. One can quickly tell that
no matter how the
sentences are rearranged, certain information does not
change. The cumulative
sums, however, almost always change. Table 8 uses
sentence 10 of the original
sample and its equivalents as an example.
Obviously, the number of words per sentence will not change no matter
where
that sentence has been rearranged in the sample. Also, the number of
types
of words (in this case, two- and three-letter words and words beginning
with
a vowel) will not change. And since the contents of the entire sample have
not been altered, the averages will remain the same, and therefore the deviations
from those averages will also stay constant. The cumulative sums, however,
de-
pend on the previous cumulative sums, which depend on the previous
cumulative
sums, etc. Anyone who has even a basic familiarity with
statistics would know
that altering the sequence almost always changes the
cumulative sums. The im-
plications of this fact are devastating for the
theory called QSUM. This should
already be apparent when comparing figures
1, 4, 5, 6, and 7 and recognizing
that they display radically different
QSUM-charts for the same utterance. Con-
trary to Farringdon's assertions,
the order of the sequence matters a great deal.
Thus one must wonder why the proponents use cumulative sums. I find no
theoretical explanation of this approach in Analysing for
Authorship beyond a pass-
ing reference to the method's origins:
"Morton first suggested the idea of cumu-
lative sum tests for language as
long ago as the 1960s, carrying the idea over
from its industrial setting:
such tests are widely used in industry as a method of
sampling averages"
(13). Farringdon is correct on this point; cumulative sums are
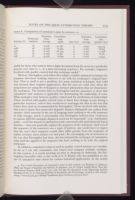
Sentence # | Words per sentence |
Deviation from average |
Cumulative sum (qsld) |
23lw+ivw | Deviation from average |
Cumulative sum (qs23lw+ivw) |
10 | 18 | −4.258 | −41.581 | 9 | −2.677 | −24.774 |
29W | 18 | −4.258 | −9.484 | 9 | −2.677 | −8.645 |
24X | 18 | −4.258 | −20.194 | 9 | −2.677 | −11.258 |
15Y | 18 | −4.258 | −15.871 | 9 | −2.677 | −8.161 |
20Z | 18 | −4.258 | 12.839 | 9 | −2.677 | 10.452 |
useful for those who want to detect slight deviations from the mean in a
particular
process over time (i. e., in a time-increasing sequence). For
example, engineers
involved with quality control find this technique
useful.[9]
Morton, Farringdon, and others thus adapt a reliable statistical technique for
purposes that have nothing whatever to do with the technique's original
func-
tion. That in itself is not a problem, for many statistical techniques
find valid
uses beyond their original applications. But the uses are valid
only when the
proponents are using the techniques to measure phenomena that
are demonstra-
bly analogous. The burden falls on Farringdon and her
associates to show that
cumulative sum analysis is applicable for
determining the authorship of texts.
What analogies exist between quality
control and the attribution of authorship?
Those involved with quality
control want to know how change occurs during a
particular sequence, and so
they would never rearrange the data in the way that
I have done (and as
recommended by Farringdon). Those involved with attribu-
tion want to know
how particular linguistic features distinguish one author from
another. Such
interests in the use of language have nothing to do with sequence
or with
change, which is presumably why Farringdon believes that "sentences
in
various QSUM examples displayed need not be sequential" (114). Attribution
study—even the branch of attribution study concerned with
quantitatively-based
theories—does not generally explore the sequence
of the sentences. And even if
the sequence of the sentences was a topic of
interest, one would have to expect
that the text's final sequence would
often differ greatly from the sequence of
earlier versions; most authors cut
and paste. By rearranging sets of sentences in
the way that Farringdon has
done, she has misused the technique of cumulative
sums and has applied it
for purposes that have nothing to do with the original
technique.
In addition, cumulative sums as used in quality control measure one variable.
As far as I can tell, cumulative sum charts never compare multiple variables.
In this context, it
is worth pointing out that in Analysing for Authorship, the
Far-
ringdons favorably refer to the work of A. F. Bissell, who has
published on the
use of cumulative sum charts for various industrial
applications. In the article
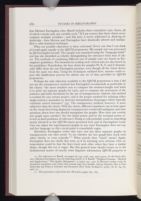
of which contain only one variable each.[10] If I am correct that these charts never
compare multiple variables—and this issue is never addressed in Analysing for
Authorship—then Morton and Farringdon have drastically altered and mishan-
dled a valid statistical technique.
What are possible objections to these criticisms? Every one that I can think
of would apply equally to the QSUM proponents. My sample text was processed
by Jill Farringdon herself. The sample was examined using the "language-habit"
test that she identified as reliably distinguishing her utterance from that
of oth-
ers. The methods of combining different sets of sample texts are
based on Far-
ringdon's guidelines. The formulas for scaling each vertical
axis are also based on
her guidelines. Nonetheless, the four charts for
samples W, X, Y, and Z dramati-
cally differ from the one Farringdon
presents, even though all the charts derive
from the same raw data. Using
the techniques outlined here, anyone could re-
peat this falsification
process for almost any set of data provided by QSUM
proponents.
Perhaps the only objection available to the QSUM proponents is that I did
not use the transparency method that Farringdon recommends as preferable to
the charts: "the more sensitive way to compare the sentence-length and habit
is to print out separate graphs for each, and to compare the movement of the
sentence and habit deviations by the use of transparencies. Indeed, this method
is essential for any serious project, and is the
proper method for isolating either
single-sentence anomalies or aberrant
interpolations of passages which typically
constitute mixed utterance" (35).
The transparency method, however, is more
subjective than the charts. With
the charts, different examiners can at least agree
on the visual data being
displayed; transparencies would add ambiguity and raise
questions about how
one should manipulate the graphs. How does one overlay
one graph upon
another? Are the initial points and/or the terminal points se-
lected as
fixed positions of reference? Doing so will probably result in something
nearly identical to the QSUM-charts presented here and in Farringdon's book.
Can one adjust the superimposed graphs in any way? Farringdon does not say,
and her language on this crucial point is remarkably vague.
Elsewhere Farringdon writes that once one has these separate graphs on
transparencies one then needs "to see whether the two graph-lines track each
other closely, or even coincide."[11]
What exactly does "track" mean? Again,
Farringdon does not clarify
this term, as if its meaning were obvious. One in-
terpretation could be
that the lines track each other when they have a similar
shape, though this
too is vague. But this general issue should return us to the
fundamental
matter of exactly what linguistic information QSUM purports to
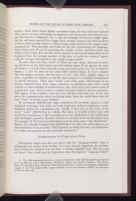
when above average information is displayed, and downward when below aver-
age information is displayed. Or, to take the example of sentence length (qsld),
the line will move upward for a longer than average sentence and downward for
a shorter than average sentence. (This average is determined by the sample under
examination.) This principle also holds for the line representing the language-
habit being used. If one is measuring the number of two- and three-letter and
initial-vowel words, then the line moves upward or downward depending on the
deviation from the average number of this class of words per sentence (again,
with the average determined by the sample being studied).
So when these two lines "track" or follow the same shape, they tend to move
up and down at the same points and with similar slopes. If one ignores the issue
of whether or not the lines coincide at any particular point, one can see
that for
figures 1–7, the two lines of each chart follow similar
shapes. In every example
that Farringdon presents, the two lines of each
chart follow similar shapes as
well—regardless of whether or not the
chart purports to establish homogenous
or mixed utterance. These lines
"track" each other quite well because of an
obvious linguistic fact: since
longer sentences, by definition, have more words
(relative to some baseline
of measurement), they will tend to have more words of
a particular class.
And of course a similar principle holds for shorter sentences.
The degree of
this tendency can vary, but will generally hold true. If one can
move the
transparencies when overlaying them, then one could show that the
lines
"track" in almost every instance.
In conclusion, QSUM uses vague definitions for its terms, misuses a valid
statistical technique, and relies on visual inspection without employing a stan-
dardized method for calculating scale. Finally, it does not, in any sense of
the
word, "work." QSUM has no validity. But since an invalid method may well
reach true conclusions, I offer no judgment on the attributions or
de-attributions
that Farringdon presents. Readers should not conclude from my discussion that,
for example, D. H. Lawrence
did indeed write the short story "The Back Road."
Rather, they should
recognize that QSUM does not offer any valid judgment on
this attribution or
on any other. This method is so faulty that one can manipulate
it to claim
any position on any particular attribution.[12]
For a brief discussion of cumulative sums in this context, see Richard A.
Johnson,
Miller and Freund's Probability and Statistics for
Engineers, 7th ed. (Upper Saddle River, New Jer-
sey: Pearson
Prentice Hall, 2005), 525–526. See also the discussion in the Engineering Statistics
Handbook of the U. S.
National Institute of Standards and Technology, located at this website:
<http://www.itl.nist.gov/div898/handbook/pmc/section3/pmc323.htm>.
For references to Bissell, see pages xii, 243, 258–259, and 315n20.
In the final refer-
ence, Michael Farringdon cites the following
article: A. F. Bissell, "Weighted Cusums—Method
and
Applications," Total Quality Management 1.3 (1990):
391–402. In Morton's earlier work, he
presented cumulative sum
charts that contain only one variable: Literary Detection:
How to Prove
Authorship and Fraud in Literature and Documents
(New York: Scribner's, 1978), 78, 81, 84, 85,
and 170.
The following article presents a critical assessment of the QSUM approach
proposed
by A. Q. Morton and S. Michaelson: Michael L. Hilton and
David I. Holmes, "An Assess-
ment of Cumulative Sum Charts for
Authorship Attribution," Literary and Linguistic
Computing 8
(1993): 73–80. The authors offer cogent
criticism, but do not attempt to falsify the technique
in the way that
I do.
![]() |
Testing QSUM Studies in bibliography | ![]() |